(Note: This is a guest post from our friends at Panoply)
Cloud-based data services are all the rage these days for many good reasons, and AWS (Amazon Web Services) is the current king of cloud-based data service providers, as this analysis carried out by StackOverflow indicates.
Two popular AWS cloud computing services for data analytics and BI are Amazon Redshift and Amazon Athena, both of which are useful for delivering actionable insights that drive better decision making from your data. However, with a dizzying amount of information available on both services, it’s a challenge to recognize what to look out for when choosing a cloud-based data service to meet your needs.
In this post, you’ll get a broad overview of cloud-based data warehousing, and you’ll come to understand the main differences between Amazon Redshift and Amazon Athena (also see this post by Panoply on the subject).
When you’re finished reading, you’ll know which service you should choose between Athena and Redshift. The comparison can also teach you what to look for in more general terms when considering any cloud-based data solution currently available.
A Data Warehouse in the Cloud
Traditional on-premise data warehouses are used for analyzing an organization’s historical data in one unified repository, pulling data from many different source systems, such as operational databases. Physical data warehouses are complex and expensive to build and maintain, though.
Cloud-based data warehouse services offer a much cheaper and easier way to use a data warehouse without needing any physical resources on site. Cloud-based providers host the necessary physical resources “in the cloud” while you simply pay for using the service.
Some examples of data warehouses in the cloud are:
- Amazon Redshift—in Redshift, you provision resources and manage those resources similar to how you would in a traditional data warehouse, without the need to host physical computing resources on-site—AWS hosts them in the cloud instead as “clusters”. You simply connect your operational data sources to the cloud and move the data into Redshift for use with analytics and BI tools. Redshift uses a customized version of PostgreSQL.
- Amazon Athena—Athena provides an interactive query service that makes it possible to directly analyze data stored in Amazon S3, which is the cloud storage service provided by AWS. You query data in Athena with standard ANSI SQL. In contrast to Redshift, Athena takes a serverless approach to data warehousing because you don’t need to provision resources or manage the infrastructure.
- Google BigQuery—BigQuery is a serverless cloud-based data analytics platform that enables querying of very large read-only datasets, and it works in conjunction with Google Storage.
- Azure SQL Data Warehouse—this Microsoft offering is a scalable and fully managed cloud-based data warehouse service.
You could write a book comparing all four of these services, so we’re going to hone in on both Amazon Redshift and Amazon Athena below.
Amazon Athena vs. Amazon Redshift – Feature Comparison
- Initialization Time: Amazon Athena is the clear winner here because you can immediately begin querying data stored on Amazon S3. Redshift, on the other hand, requires you to prepare a cluster of computing resources and load data into the tables you create.
- User-defined Functions: Amazon Redshift supports user-defined functions (UDFs), which are procedures that accept parameters, perform an action, and return the result of that action as a value. Amazon Athena has no support for UDFs.
- Data Types: Amazon Athena supports more complex data types, such as arrays, maps, and structs, while Redshift has no support for such complex data types.
- Performance: For basic table scans and small aggregations, Amazon Athena outperforms Redshift. However, for complex joins and larger aggregations, Redshift is a better option.
- Cost: Athena’s cost is based on the amount of data scanned in each query, which means it’s important to compress and partition data. Since Amazon Athena queries data on S3, the total cost of S3 data storage combined with Athena query costs gives the full price. Redshift’s cost depends on the type of cloud instances used to build your cluster, and whether you want to pay as you use (on demand) or commit to a certain term of usage (reserved instances).
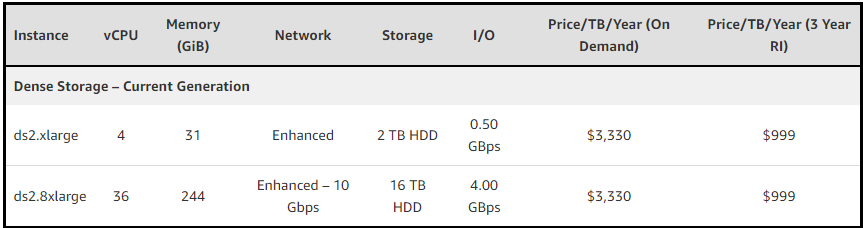
Athena’s cost is $5 per terabyte of data scanned, while Redshift’s hourly costs range from $0.250 to $4.800 per hour for a DC instance, and $0.850 to $6.800 per hour for a DS instance.
AWS Redshift Spectrum, Athena, S3
Redshift Spectrum is a powerful feature that enables data querying in Redshift directly from S3. With Spectrum you can create a read-only external table, with its data located in a specified S3 path, and immediately begin querying that data without inserting it into Redshift. You can also join the external tables with tables that already reside on Redshift.
Querying data in S3: sounds familiar, right? That’s because Amazon Athena performs a similar function—it’s an S3 querying service. It’s important to note, however, that Spectrum is not an integration between Redshift and Athena—Redshift queries the relevant data on its own from S3 without the help of Athena.
If you are already an Amazon Redshift user, it makes sense to opt for Spectrum over Athena because of the convenience. However, if you aren’t currently using Redshift, it’s best to choose Athena over Spectrum because your investment in computing resources might go underutilized in Redshift. For your current analytics needs, Athena is likely to do the job—you can always invest in a Redshift+Spectrum combination later on when it’s needed to handle lots of data.
Athena vs. Redshift – Which Should You Choose?
There is no widespread consensus on whether Amazon Athena is better than Redshift or vice versa—both services suit different uses.
- Amazon Athena is much quicker and easier to set up than Redshift, and this querying service outperforms Redshift on all basic table scans and small aggregations. The accessibility of Athena makes it better suited to running quick ad hoc queries.
- For complex joins, larger aggregations, and very large datasets, Redshift is better due to its computational capacity and highly scalable infrastructure.
- The conclusion, therefore, is that Redshift is likely the cloud-based data warehouse platform of choice if you have lots of data and many complex queries to run. Amazon Athena is the recommended cloud-based service for analyzing your data in all other cases, and it’s suitable for small- to medium-sized organizations.
Closing Thoughts
Cloud-based data warehouses are quickly replacing traditional on-premise data warehouses because of their convenience, lower cost, and scalability.
Amazon Athena and Amazon Redshift take differing approaches to cloud-based data analytics services—Redshift requires resource provisioning and infrastructural management while Athena abstracts operational management away from users and allows direct querying of data stored on Amazon S3.
Amazon Spectrum provides separation of storage and compute in Redshift by allowing you to directly query data in S3, similar to Athena. Spectrum is useful if you already use Redshift, but you shouldn’t base your decision on Athena versus Redshift on the Spectrum feature.
The relevant comparison between Amazon Athena and Redshift relates to how they perform, what they cost, which tools they support, their usability, their accessibility, supported data types, and user-defined functions. You should base your end decision between Redshift and Athena on these factors, prioritizing the most important aspects of each service for your particular business. Maybe you prefer Athena’s effortless accessibility? Or maybe you’d rather the control and scalability you get in Redshift?
When weighing up any potential cloud-based data warehouse, always consider the above factors, instead of just choosing the most affordable solution.